Good data is more important than big data in developing digital biomarkers. Big data is often sold as the solution to all digital data analyses and is touted to revolutionize healthcare in the coming years. However, the problem with a superabundance of data is that digital biomarker development becomes a fishing expedition, and the catch may not be relevant to the question. Without a hypothesis (often the case in biomarker development with big data), accurate results may be too low to use in a clinical trial or, even worse, divergent with no findings.
That said, big data does have its place in improving biomarker algorithms and the results that advance the accuracy and repeatability of the endpoint. In this article, we will explain why good data is required to ensure validated digital biomarkers for clinical trials and provide examples of how we ensure “good” data is obtained from wearable sensors.
Requirements of Good Data
There are three essential elements of good data within the biomarker development framework.
- 1. Validation datasets with labeled ground truth. Big data attempts to overcome the requirements for good data by applying advanced analytics to extensive datasets. For example, big data techniques do not involve traditional validation approaches. Instead, they employ analytics such as “deep learning” or AI, which use the data itself to learn important patterns and infer important outcomes with little or no human input or corresponding ground truth data.
- 2. Real-world data cleaned to remove signal artifact. Cleaning unwanted signal artifact from wearable sensor signals requires effort and expertise. Big data adds more data to the equation instead of focusing on clean sensor signals, reducing the level of accuracy and precision needed to identify a treatment effect. In clinical trials, patient recruitment and retention are difficult, and simply adding more and more data is not possible (and very expensive).
- 3. Hypothesis-driven, clinically meaningful events identified and extracted from the sensor. Scientists often get caught up in letting the data or technology drive the question. Data is being amassed in unprecedented amounts, but this should not dictate the science. Hypothesis-driven research and sound scientific methodology are vital and require good data is used in development.
Steps to Identifying Good Data
We use a three-step process to identify and analyze the good wearable sensor data. Each step ensures that we keep the hypothesis in mind and understand that one size does not fit all in clinical trial research.
Step 1: Identify the Fit for Purpose of the Wearable Sensor.
The first step to ensure digital biomarker development is driven by useful quality data is to collect good data. The most important aspect of this is fit for purpose: the wearable sensor that is being used in a clinical trial and to develop a novel endpoint must be able to provide the quality and resolution of data needed to find the hypothesis endpoint.
Example: We help clinical researchers understand inflammatory and stress-related symptoms by providing endpoints of Heart Rate Variability (HRV) measures using wrist-based PPG (pulse oximeters) or patch-worn ECG sensors. Because HRV is a measure of variability in the beat-to-beat timing, it’s particularly suspectable to bad data; any noise will increase variability and result in an incorrect evaluation.
The choice of a wrist-worn PPG is typically appropriate for measures during sleep and or specific low-activity events over long durations. A patch-worn ECG provides higher-quality data during activity. However, it’s harder to maintain during long-term wear, making it more appropriate for activity or rehabilitation endpoints with short-period wear.
Step 2. Manage the Wearable Sensor Noise.
Noise in the data can be easily misidentified as an event, and developing a digital biomarker from poor-quality data will inevitably fail. Managing noise and checking for missing data is critical to developing an endpoint. It ensures that only good data is analyzed.
VivoSense® data analysis platform’s cleaning algorithms and AI are invaluable for examining multiple data channels (from any wearable source) for noise and potential artifacts. The human-augmented AI approach described below can then further improve the quality of the data.
Example: An increase in HR may be detected in a subject when their grandchild bumps their ECG patch during play. The data could easily be falsely identified as a disease-specific event. Closer inspection may find that the subject’s increased HR is associated with higher activity. Incorrectly identified RWave peaks could also contribute to a noisy ECG signal. VivoSense® algorithms identify these regions and manage them. If the noise is of a short period, you can use interpolation; if it is longer, you can exclude it.
Step 3. Labeling Events and Human Augmented AI.
After the noise in the data has been managed, we can begin to identify the disease-specific events and label them appropriately. The good data approach requires that events are accurately labeled so that valid endpoints can be defined.
Typically, big data-tuned AI can produce a receiver operator characteristic (ROC) of 0.8 or around 80% accuracy to balance between True Positive and False Positive rates. With Human Augmented AI, we tune our AI and algorithms to increase both the True positive and False positive rate to improve ROC and accuracy, which is essential for good data. An expert human reviewer is then able to quickly review all events and remove false positives. VivoSense® gets the process done very quickly, reducing the human review of 24 hours of data to a matter of minutes and ensuring high-quality data is used for digital biomarker development. The result facilitates:
- • Improved accuracy
- • Reduction in the required digital endpoint effect
- • Reduction in subject sample size
- • Providing evidence for validation
- • Better Science and Improved outcomes
Artifact Management is Crucial for Good Data
Our data experts are often consulted on how to analyze wearable sensor data after it has been collected. In most cases, the problem is the quality of the data collected. Wearable sensor data is inherently noisy for the simple reason that the data are collected during real-world activities. An ECG patch worn during daily activity will suffer from motion artifact, even if it is of high quality and resolution and has been correctly applied. A wrist-worn PPG or actigraphy sensor will have difficulty accurately measuring heart rate during an activity, such as walking.
If the noise in these data is not managed, the results may be too variable or the output misunderstood. To provide the accuracy needed to develop a digital biomarker for clinical trials and further healthcare applications, we must understand and manage this noise. By doing this, we can analyze the Good data and validate the new endpoints.
Good data is more important than Big data to us in developing novel digital endpoints and producing evidence for validation.
In our eBook, 4 Key Principles of Digital Biomarker Discovery, we expand on each of the four principles of digital biomarker discovery and provide specific examples from our own work and literature when appropriate. We will also explain how and why these principles are most impactful and relevant when biomarker development is conducted in early-stage clinical trials or even earlier pilot studies.
Dudley Tabakin
Dudley Tabakin, MSc. is Chief Executive Officer and co-founder of VivoSense and a fervent believer in “good data” over “big data” in the development of digital endpoints from wearable sensor technology.
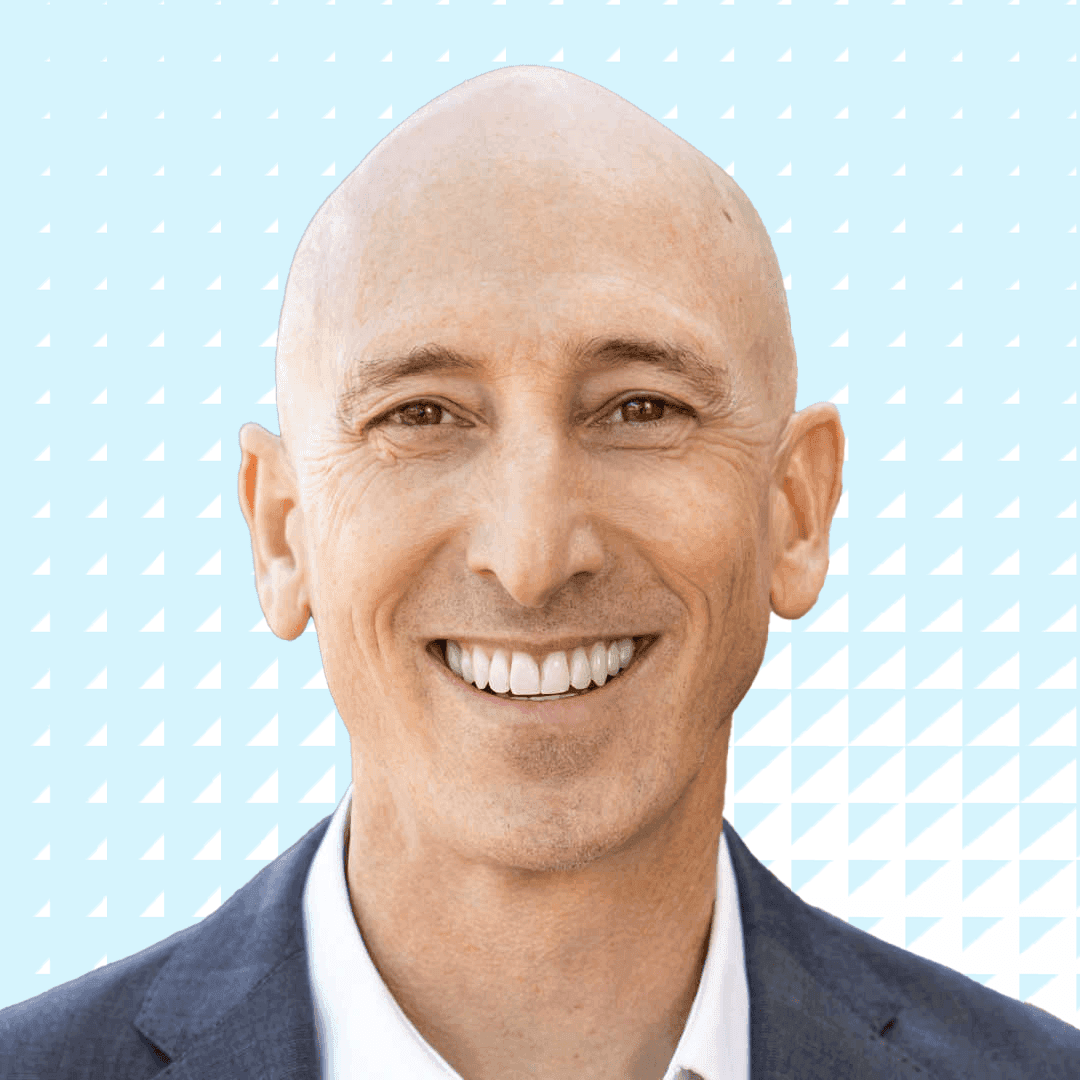